Mixed Pixel Snow Cover Mapping of Hyperspectral Imagery using Linear Spectral Unmixing Method
Traditionally, snow cover mapping has been a binary affair, categorizing pixels as either snow or non-snow. However, this approach oversimplifies the complexity of snow-covered landscapes, where mixed pixels—combinations of snow, vegetation, and soil—abound. In this study, conducted in the lower Indian Himalayan region during February 2017, we delve into the intricacies of snow reflectance and mixed pixels using Hyperion hyperspectral imagery. By employing the Linear Spectral Unmixing (LSU) method, we aim to enhance the accuracy and efficiency of snow cover estimation at a finer, mixed pixel level.
Field experiments utilizing an ASD Spectroradiometer (350-2500nm) were conducted in Dhundi, Himachal Pradesh, India, to capture ground truth data. Snow, vegetation, and soil reflectance spectra were meticulously collected, forming the basis for end-member spectra identification. Our approach involves predicting these end-member spectra for each pixel, allowing for the unmixing of mixed pixels using a linear mixture model.
In addition to examining the effects of snow reflectance and mixed pixels, this study encompasses comprehensive data processing steps. Dimensionality reduction techniques and corrections for atmospheric and topographic distortions in Hyperion hyperspectral data are analyzed, ensuring the accuracy of our results.
Preliminary findings suggest that our novel method not only improves accuracy but also reduces computation time. By accurately estimating the actual snow cover region at the mixed pixel level, our approach offers a valuable tool for researchers and policymakers involved in snow monitoring and management.
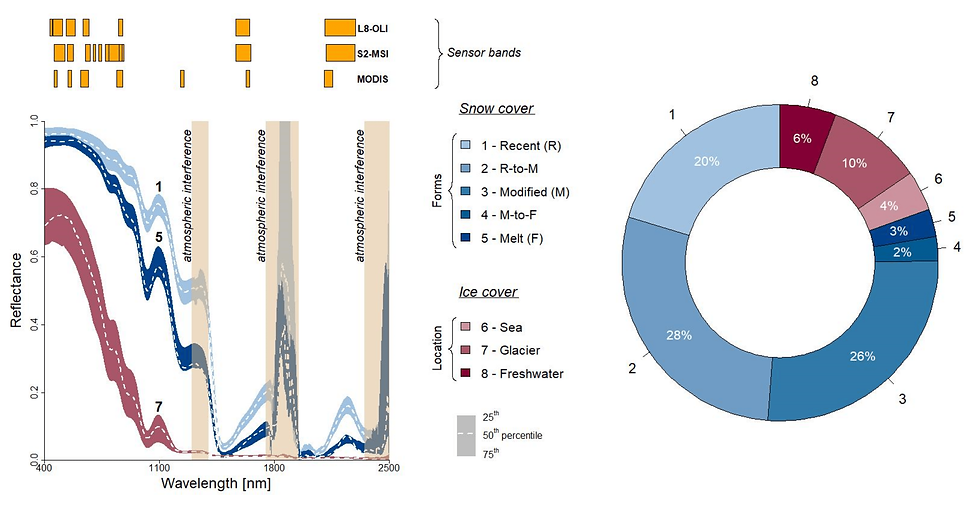
Introduction
Snow cover mapping is crucial for various applications, including climate modelling, hydrological assessments, and disaster management. Conventional methods typically classify pixels as either snow-covered or not, overlooking the intricate mixture of surface types present in many landscapes. This oversimplification can lead to inaccuracies, particularly in regions with heterogeneous terrain and vegetation.
Hyperspectral imagery, with its ability to capture a wide range of wavelengths, offers a promising solution for improving snow cover mapping. By leveraging the wealth of spectral information contained in hyperspectral data, researchers can better discriminate between snow, vegetation, and soil, even within mixed pixels.
Methodology
The Linear Spectral Unmixing (LSU) method forms the backbone of our approach. By identifying end-member spectra for snow, vegetation, and soil, we can effectively unmix mixed pixels using a linear combination model. Field experiments were conducted to collect ground truth data using an ASD Spectroradiometer, ensuring the accuracy of our end-member spectra.
Additionally, to mitigate atmospheric and topographic distortions inherent in hyperspectral imagery, we employed advanced data processing techniques. Dimensionality reduction methods such as Principal Component Analysis (PCA) were utilized to streamline the analysis, while corrections for atmospheric effects and terrain variations were applied to enhance the accuracy of our results. Results and Discussion
Preliminary results indicate that our method outperforms traditional snow cover mapping techniques in terms of accuracy and computational efficiency. By accounting for mixed pixels and leveraging hyperspectral data, we achieve higher precision in delineating snow-covered regions. Furthermore, our approach provides valuable insights into the spectral characteristics of snow, vegetation, and soil, enhancing our understanding of these complex landscapes.
Conclusion
In conclusion, the application of Linear Spectral Unmixing (LSU) to hyperspectral imagery holds great promise for advancing snow cover mapping capabilities. By accurately estimating snow cover at the mixed pixel level, our method offers a more nuanced understanding of snow-covered landscapes. Moving forward, further refinements and validation efforts will be undertaken to ensure the reliability and applicability of our approach across diverse environments.
Comments